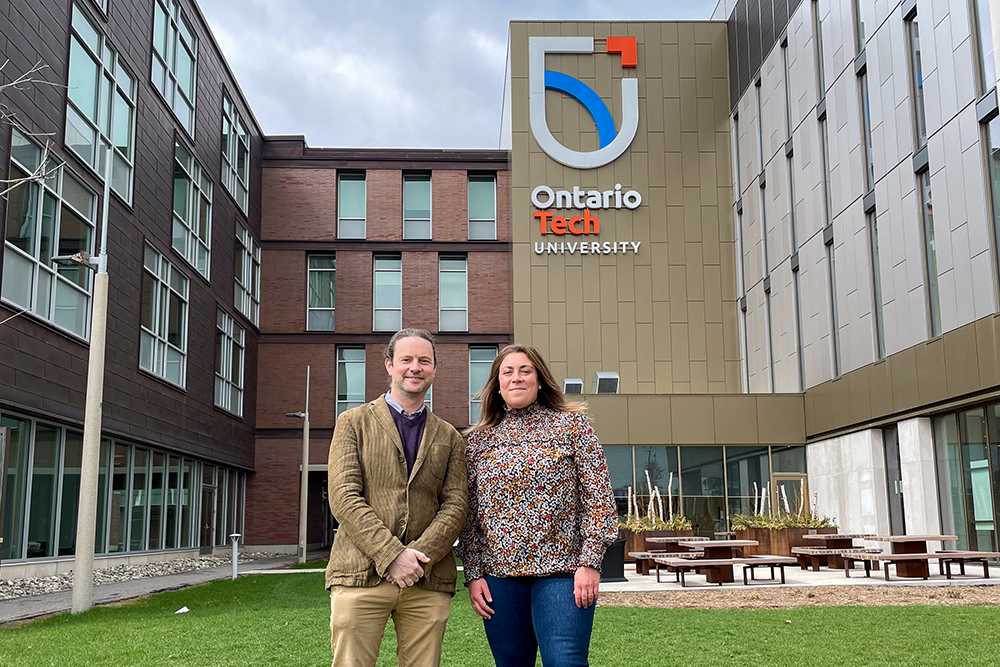
Crime scene investigations require reliable forensic science tools to make air-tight cases, eliminating any potential for ambiguity or doubt. But current methods used to interpret evidence at crime scenes have been criticized globally for subjectivity and lack of scientific rigour.
One area of criticism is the existing classification schemes of bloodstain pattern analysis (BPA), which:
- Sometimes lack clarity concerning the cause and formation of the bloodstain.
- Are not conserved as the environment varies.
- Have issues dealing with feature ambiguity.
Some branches of forensic science have modified existing schemes in response; but more recently, fundamental issues with the assumptions behind these classification schemes have been exposed.
To address these issues, an Ontario Tech University-led research team has been awarded Government of Canada Interagency research funding through the New Frontiers in Research Fund (NFRF) Exploration stream in a project entitled ‘Reimagining forensic BPA from the bottom up’.
The Ontario Tech research will be led by Principal Investigator Dr. Theresa Stotesbury, Assistant Professor (Forensic Science), Faculty of Science and Dr. Peter Lewis, Associate Professor (Computer Science), Faculty of Business and Information Technology and Canada Research Chair in Trustworthy Artificial Intelligence (Co-Principal Investigator).
The interdisciplinary research project includes collaborators:
- Cecilia Hageman, Assistant Professor and Undergraduate Program Director Forensic Science, Faculty of Science, Ontario Tech University
- Caitlin Pakosh, JD, Assistant Professor, Forensic Science, University of Toronto Mississauga
- Amanda Lowe, Forensic Research and Training Analyst within Forensic Identification Services, Ontario Provincial Police
The project is valued at $250,000 over two years.
About the bloodstain pattern analysis research
The team will take a data-driven approach, unburdened by the assumptions in historical schemes, to produce a bloodstain pattern analysis tool aimed at helping and guiding everyone connected to the criminal justice system in a transparent and interpretable way. The tool will be underpinned by a new classification scheme that integrates both data-driven insights and forensic expertise, and captures a more rigorous, accurate, and informed understanding of the causes of bloodstains.
The research team will use unsupervised machine learning, a family of techniques that analyzes untagged data, and facilitates the emergence of natural patterns, which may otherwise remain hidden or obscured by human bias. This approach is completely different from supervised learning, already in use in BPA, where assumptions from historical taxonomies form the basis of classification, rather than emerge from the data.
Unsupervised learning algorithms have contributed to significant advances in a wide range of disciplines from medical diagnosis to marketing, revolutionizing previous manual processes based solely on expertise, experience and intuition. A wide range bloodstain patterns from indoor and outdoor environments will examine if BPA data can enable a similar revolution in data-driven forensic science.
Quotes
“I’m really looking forward to working with our team on this research. I think we will have a real impact on improving the rigour, understanding and communication in this exciting field of forensic science.”
-Dr. Theresa Stotesbury, Assistant Professor (Forensic Science), Faculty of Science, Ontario Tech University
“The importance of building models that are trustworthy cannot be overstated in applications in the criminal justice system. Integrating explainable and interpretable machine learning methods with domain expertise are essential to this research. The potential impact in reducing wrongful convictions, as well as the interdisciplinary nature of this project and the partnerships that have been built to deliver it, make this a particularly exciting area of research to work in.”
-Dr. Peter Lewis, Associate Professor (Computer Science), Faculty of Business and Information Technology, and Canada Research Chair in Trustworthy Artificial Intelligence, Ontario Tech University
Media contact
Bryan Oliver
Communications and Marketing
Ontario Tech University
289.928.3653 (mobile)
bryan.oliver@ontariotechu.ca